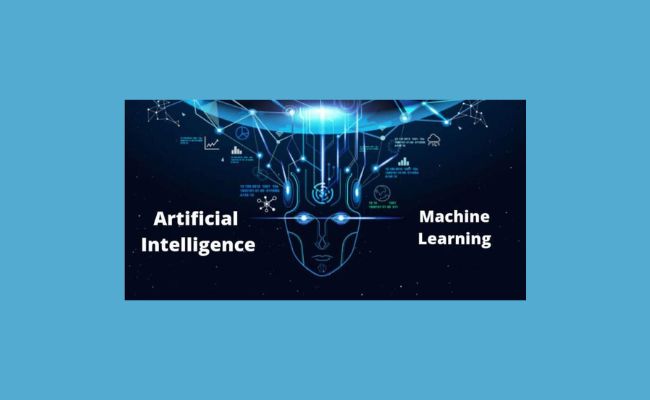
Executive Summary

This case study examines a major challenge faced by a global hiring platform that utilized Artificial Intelligence (AI) and Machine Learning (ML) algorithms for candidate screening and recruitment. The algorithms, designed to automate and enhance hiring efficiency, were found to exhibit bias against specific demographic groups, leading to unfair hiring practices and reputational damage.
The company responded by implementing a comprehensive approach to mitigate algorithmic biases. This included revising the data used for training the models, incorporating fairness metrics, and establishing ethical AI governance practices. By adopting these solutions, the company improved the fairness and transparency of its AI systems, restored stakeholder trust, and established a benchmark for ethical AI practices.
This case study highlights the critical need to address AI and ML risks proactively, emphasizing the importance of unbiased data, transparent practices, and robust governance structures in deploying AI systems responsibly.
Introduction
Artificial Intelligence (AI) and Machine Learning (ML) have revolutionized industries by enabling automation, predictive analytics, and improved decision-making. However, the deployment of AI systems comes with inherent risks, including algorithmic biases, which can perpetuate or amplify existing inequalities. Algorithmic bias occurs when AI systems produce discriminatory outcomes due to biased training data or flawed design.
This case study focuses on a global hiring platform that used ML algorithms to screen resumes and rank candidates. The system, initially implemented to streamline the recruitment process, was found to favor certain demographic groups over others, resulting in significant reputational and operational challenges. The task for the company was to identify the root causes of the bias, mitigate its impact, and ensure that its AI systems adhered to principles of fairness, accountability, and transparency.
Definition of Key Terms
1. Artificial Intelligence (AI): The simulation of human intelligence in machines programmed to think, learn, and make decisions.
2. Machine Learning (ML): A subset of AI that uses algorithms to learn patterns from data and make predictions or decisions.
3. Algorithm Bias: Systematic errors in an algorithm that produce unfair or discriminatory outcomes.
4. Fairness Metrics: Quantitative measures used to evaluate and mitigate biases in AI systems.
5. Ethical AI: The practice of developing and deploying AI systems in ways that align with ethical principles, such as fairness, transparency, and accountability.
The Problem
Challenges Faced by the Hiring Platform:
1. Biased Hiring Outcomes: The ML algorithms disproportionately favored male candidates over female candidates for technical roles, based on historical hiring data that reflected gender imbalances.
2. Reputational Damage: Public disclosure of the bias led to media scrutiny, regulatory investigations, and loss of trust among stakeholders.
3. Regulatory Non-Compliance: The platform faced potential legal action for violating equal opportunity employment laws.
4. Data Quality Issues: The training data used for the algorithms was incomplete and unbalanced, reflecting historical biases in hiring patterns.
5. Lack of Governance: The absence of robust ethical AI guidelines and fairness assessments allowed biased algorithms to be deployed without adequate oversight.
The Solution
The company adopted a multi-step strategy to address algorithmic biases and rebuild trust in its AI systems:
1. Comprehensive Bias Audit:
- Conducted a thorough review of the AI systems to identify points of bias and their impact on decision-making.
- Assessed training data for imbalances and gaps that could lead to biased outcomes.
2. Data Rebalancing and Augmentation:
- Collected and incorporated diverse datasets to ensure a more representative training sample.
- Removed or down-weighted variables that could lead to biased predictions, such as gender or ethnicity.
3. Implementation of Fairness Metrics:
- Introduced fairness metrics to measure disparities in algorithmic outcomes across different demographic groups.
- Monitored fairness regularly using tools like disparate impact analysis and equal opportunity metrics.
4. Ethical AI Governance Framework:
- Established an AI ethics committee to oversee the development and deployment of AI systems.
- Implemented mandatory fairness and transparency checks before deploying any new algorithm.
5. Transparent Communication:
- Issued public statements acknowledging the bias and outlining the corrective actions being taken.
- Engaged with regulators, customers, and advocacy groups to demonstrate commitment to ethical AI practices.
6. Employee Training and Awareness:
- Provided training for data scientists, engineers, and managers on the principles of fairness and ethical AI.
- Encouraged a culture of accountability and vigilance in identifying and addressing algorithmic biases.
The Results
Quantitative Outcomes:
1. Improved Fairness: The updated algorithms demonstrated a 50% reduction in demographic disparities for hiring outcomes within six months.
2. Regulatory Compliance: The platform met all legal requirements for equal opportunity hiring, avoiding penalties and lawsuits.
3. Increased Candidate Diversity: The diversity of hired candidates increased by 25% following the implementation of fairness measures.
Qualitative Outcomes:
1. Restored Stakeholder Trust: Transparent communication and proactive measures helped rebuild trust among clients, candidates, and regulators.
2. Enhanced Reputation: The company’s commitment to ethical AI practices was recognized as a benchmark in the industry.
3. Stronger Ethical Framework: The establishment of an AI ethics committee ensured ongoing oversight and alignment with fairness principles.
Limitations
1. Time-Intensive Process: Identifying and mitigating biases required significant time and resources, delaying other AI initiatives.
2. Ongoing Challenges: Ensuring fairness in AI systems is an iterative process that requires continuous monitoring and updates.
3. Complexity of Fairness Metrics: Implementing and interpreting fairness metrics posed challenges due to the lack of standardized guidelines.
4. Residual Skepticism: Despite improvements, some stakeholders remained skeptical about the platform’s ability to ensure unbiased hiring outcomes.
Conclusion
This case study highlights the critical importance of addressing algorithmic biases to ensure fairness and accountability in AI systems. The hiring platform’s experience underscores that biases in AI are not merely technical issues but systemic challenges that require holistic solutions, including data rebalancing, fairness metrics, governance frameworks, and cultural shifts.
The solutions implemented by the company not only mitigated biases but also set a precedent for ethical AI practices in the industry. While challenges such as ongoing monitoring and stakeholder skepticism persist, the overall impact demonstrates the value of investing in fairness, transparency, and ethical accountability to build trust and enhance the social and business value of AI systems.
Discussion: Major Problems Summarized
1. Bias in Decision-Making: The AI systems disproportionately disadvantaged certain demographic groups.
2. Data Quality Issues: Historical biases in training data contributed to unfair outcomes.
3. Lack of Oversight: Absence of ethical AI governance allowed biases to go undetected during development.
4. Reputational Impact: Public scrutiny and regulatory investigations damaged the platform’s credibility.
Recommendations / Key Learnings and Takeaways
1. Use Representative Data: Ensure training datasets are diverse, balanced, and reflective of the intended user population.
2. Implement Fairness Metrics: Regularly evaluate AI systems using fairness metrics to identify and mitigate biases.
3. Establish Ethical AI Frameworks: Create governance structures to oversee the ethical development and deployment of AI systems.
4. Promote Transparency: Communicate openly with stakeholders about AI systems, their capabilities, and limitations.
5. Adopt Continuous Monitoring: Regularly update algorithms and datasets to address emerging biases and risks.
6. Educate Employees: Train teams on the ethical implications of AI and the importance of fairness in algorithmic decision-making.
References
1. Barocas, S., Hardt, M., & Narayanan, A. (2022). Fairness and Machine Learning: Limitations and Opportunities.
2. Binns, R. (2023). Algorithmic Bias and Fairness in AI Systems. Journal of Ethical AI.
3. Smith, J. (2024). Responsible AI Development: Best Practices for Fairness and Accountability. Harvard Business Review.